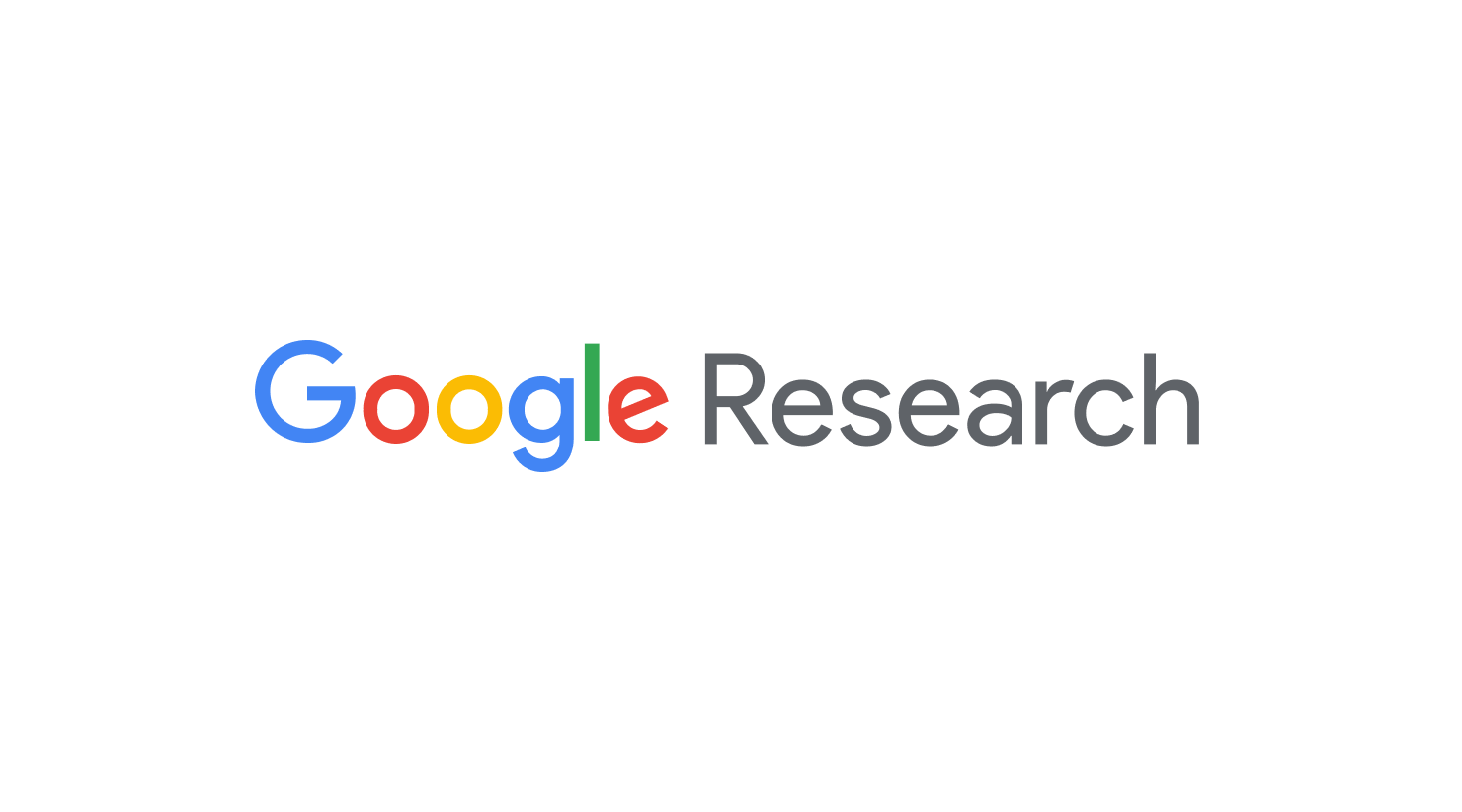
On the predictability of Search Trends
August 17, 2009
Posted by Yossi Matias, Niv Efron, and Yair Shimshoni, Google Labs, Israel.
Since launching Google Trends and Google Insights for Search, we've been providing daily insight into what the world is searching for. An understanding of search trends can be useful for advertisers, marketers, economists, scholars, and anyone else interested in knowing more about their world and what's currently top-of-mind.
As many have observed, the trends of some search queries are quite seasonal and have repeated patterns. See, for instance, the search trends for the query "ski" hit their peak during the winter seasons in the US and Australia. The search trends for basketball correlate with annual league events, and are consistent year-over-year. When looking at trends of the aggregated volume of search queries related to particular categories, one can also observe regular patterns in some categories like Food & Drink or Automotive. Such trends sequences appear quite predictable, and one would naturally expect the patterns of previous years to repeat looking forward.
On the other hand, for many other search queries and categories, the trends are quite irregular and hard to predict. Examples include the search trends for obama, twitter, android, or global warming, and the trend of aggregate searches in the News & Current Events category.
Having predictable trends for a search query or for a group of queries could have interesting ramifications. One could forecast the trends into the future, and use it as a "best guess" for various business decisions such as budget planning, marketing campaigns and resource allocations. One could identify deviation from such forecasting and identify new factors that are influencing the search volume as demonstrated in Flu Trends.
We were therefore interested in the following questions:
- How many search queries have trends that are predictable?
- Are some categories more predictable than others? How is the distribution of predictable trends between the various categories?
- How predictable are the trends of aggregated search queries for different categories? Which categories are more predictable and which are less so?
Specifically, we have used a simple forecasting model that learns basic seasonality and general trend. For each trends sequence of interest, we take a point in time, t, which is about a year back, compute a one year forecasting for t based on historical data available at time t, and compare it to the actual trends sequence that occurs since time t. The error between the forecasting trends and the actual trends characterizes the predictability level of a sequence, and when the error is smaller than a pre-defined threshold, we denote the trends query as predictable.
Our work to date is summarized in a paper called On the Predictability of Search Trends which includes the following observations:
- Over half of the most popular Google search queries are predictable in a 12 month ahead forecast, with a mean absolute prediction error of about 12%.
- Nearly half of the most popular queries are not predictable (with respect to the model we have used).
- Some categories have particularly high fraction of predictable queries; for instance, Health (74%), Food & Drink (67%) and Travel (65%).
- Some categories have particularly low fraction of predictable queries; for instance, Entertainment (35%) and Social Networks & Online Communities (27%).
- The trends of aggregated queries per categories are much more predictable: 88% of the aggregated category search trends of over 600 categories in Insights for Search are predictable, with a mean absolute prediction error of of less than 6%.
- There is a clear association between the existence of seasonality patterns and higher predictability, as well as an association between high levels of outliers and lower predictability. For the Entertainment category that has typically less seasonal search behavior as well as relatively higher number of singular spikes of interest, we have seen a predictability of 35%, where as the category of Travel with a very seasonal behavior and lower tendency for short spikes of interest had a predictability of 65%.
- One should expect the actual search trends to deviate from forecast for many predictable queries, due to possible events and dynamic circumstances.
- We show the forecasting vs actual for trends of a few categories, including some that were used recently for predicting the present of various economic indicators. This demonstrates how forecasting can serve as a good baseline for identifying interesting deviations in actual search traffic.
There are many more questions that can be looked at regarding search trends in general, and their predictability in particular, including design and testing more advanced forecasting models, getting other insights into the distributions of sequences, and demonstrating interesting deviations of actual-vs-forecast for predictable trends series. We'd love to hear from you - share with us your findings, published results or insights - email us at insightsforsearch@google.com.